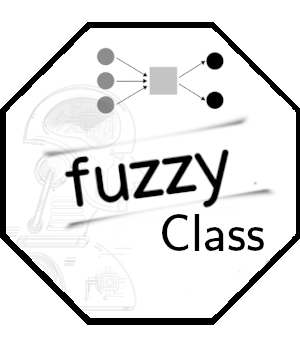
Double Weighted Fuzzy Gamma Naive Bayes
Source:R/DWFuzzyGammaNaiveBayes.R
DWFuzzyGammaNaiveBayes.Rd
DWFuzzyGammaNaiveBayes
Double Weighted Fuzzy Gamma Naive Bayes
Arguments
- train
matrix or data frame of training set cases.
- cl
factor of true classifications of training set
- cores
how many cores of the computer do you want to use to use for prediction (default = 2)
- fuzzy
boolean variable to use the membership function
- wdelta
vector weight each class
- weta
vector weight each feature
References
Moraes RM, Soares EAMG, Machado LS (2020). “A double weighted fuzzy gamma naive bayes classifier.” Journal of Intelligent & Fuzzy Systems, 38(1), 577--588.
Examples
set.seed(1) # determining a seed
data(GamWeightData)
# Splitting into Training and Testing
split <- caTools::sample.split(t(GamWeightData[, 1]), SplitRatio = 0.7)
Train <- subset(GamWeightData, split == "TRUE")
Test <- subset(GamWeightData, split == "FALSE")
# ----------------
# matrix or data frame of test set cases.
# A vector will be interpreted as a row vector for a single case.
test <- Test[, -4]
fit_NBT <- DWFuzzyGammaNaiveBayes(
train = Train[, -4],
cl = Train[, 4], cores = 2,
wdelta = c(2.002/6,1.998/6,2.000/6),
weta = c(3/10,2/10, 5/10)
)
pred_NBT <- predict(fit_NBT, test)
head(pred_NBT)
#> [1] 1 1 1 1 1 1
#> Levels: 1 2 3
head(Test[, 4])
#> [1] 1 1 1 1 1 1
#> Levels: 1 2 3